This post is part of our ongoing Digital Spark series. Be sure to also check out the series introduction, as well as the other posts in the series: setting digital health apart, health systems strengthening, embracing collaboration, and lessons from COVID-19.
The field of digital health is rapidly changing.
As we begin seeing more and more rollouts of national-scale digital health programs around the world, we also see the focus of global digital health shifting from proof-of-concept pilots to health systems strengthening initiatives. Such initiatives, when implemented responsibly, demonstrate great potential for collaboration and long-term sustainability and offer exciting opportunities to transform not only how health systems function, but also how people access and receive care.
Nonetheless, these projects are highly complex and necessarily involve government and non-government partners with diverse sets of skills and competencies. Under the surface of these projects lie the personal, individual-level data of beneficiaries accessing digitally enabled services—after all, without digital data, there is no digital health—and as the amount of data produced by digital health interventions comes with opportunities and risks, questions begin to arise about how best to handle that data.
It is within this climate that we must discuss data protection and privacy (DPP). At D-tree, we feel that we have an undeniable responsibility to use data in a way that does no harm and reduces the risks to which the “data subjects” (people in communities) are exposed. At the same time, and within the constraints of ethical data use, we also have the responsibility to use data to improve the health systems and services where this data is produced. This balancing of responsibilities touches on many issues related to data ethics, with DPP as one that is particularly important for the following reasons:
(i) DPP is relevant in any context where information identifying individuals or groups is processed, digital or not, health data or other.
For example, the information “John Doe is the president of the Central Region football fan club,” which seems perfectly inconspicuous or harmless, is a piece of personal information that can be used to identify him and that should therefore be protected. Imagine that John Doe’s fan club had a visible role in a social movement that is then classified as a criminal movement by the political rulers. Because John Doe’s record includes his association with the football club, anyone can easily identify him—leaving John exposed to potential risk or harm. The evaluation of whether something is inconspicuous or harmless is normally not straightforward and can change over time.
(ii) Contexts like health are often considered particularly sensitive.
Depending on cultural or political norms, some health-related information can lead to social stigma, discrimination, or physical harm. Regardless of whether one subscribes to the notion that some health data are more sensitive than others, or to the notion that health data itself is more sensitive than other types of data, we need to analyze the risk and impact of the disclosure of personal or group information; after all, disclosure of personally or demographically identifiable health information could have a negative impact on people’s lives if this data were accessed by those without authorization.
(iii) When information is processed digitally, the scale of data, as well as how those data are interlinked, become additional concerns.
In a non-digital dataset, as is the case with paper medical records, combining patient records and reviewing past information is clunky, time consuming, and disjointed. However, when these records are digitized, we can quickly compile a list of all individuals with a certain characteristic or look up whether a person had a certain health condition in the past. We might have longitudinal data about individuals that can be linked through a unique identifier (which is increasingly common in digital health programs and important for providing ongoing care), making it much easier to draw on medical histories to make inferences about a current illness or to identify previously unidentified individuals or groups. While these aspects of digital datasets are impactful in striving for continuity of care, DPP comes to the forefront as a potential serious concern.
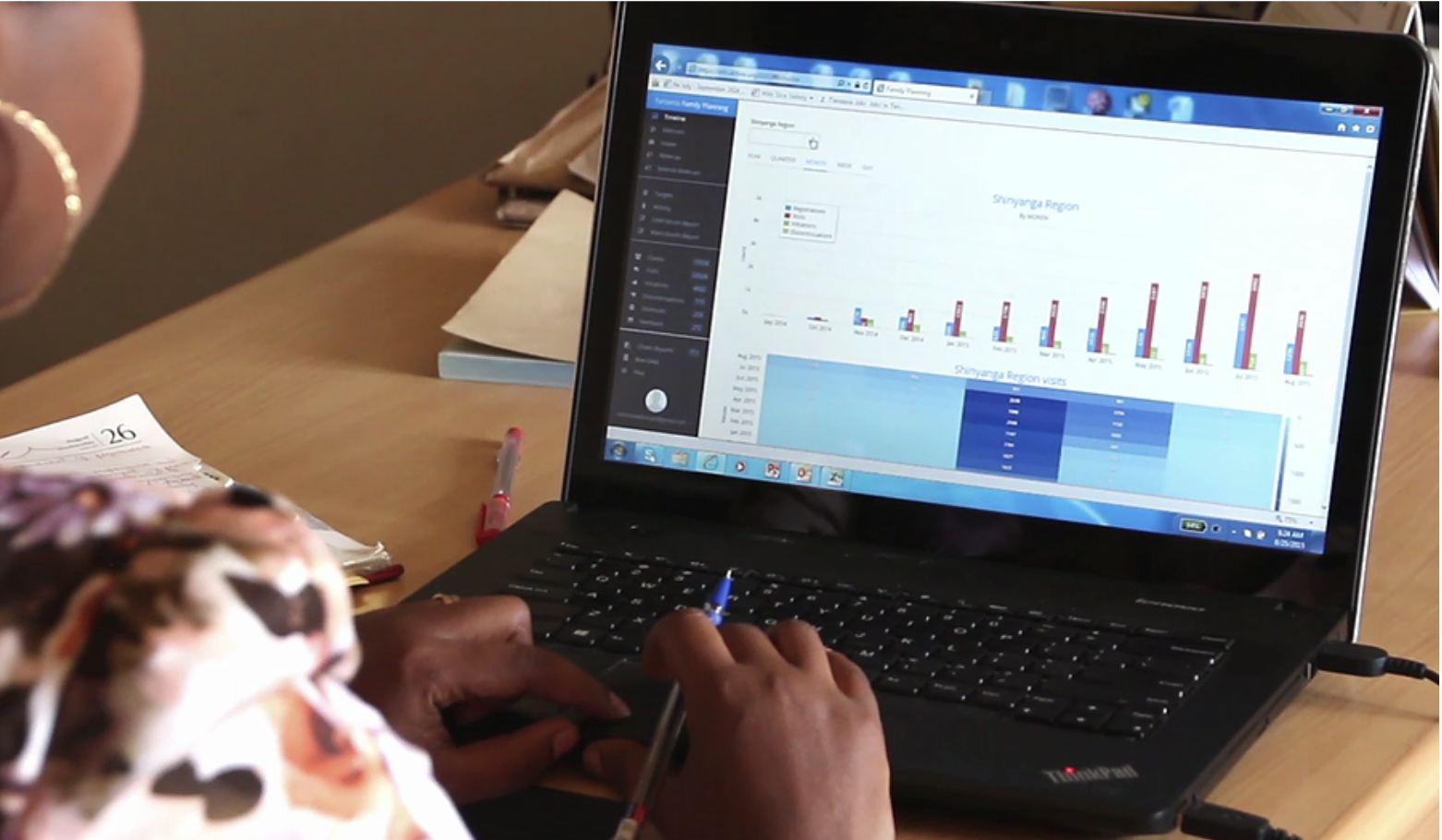
(iv) Although laws, regulations, policies or principles around DPP might be available, it is often not straightforward how to implement them.
It is often difficult to translate laws and regulations, as well as organizational policies and principles, into processes, workflows and technology. Many countries do not have comprehensive data protection policies, and when they do exist, guidance about how to protect data often lacks the specificity for direct application to a given project without significant additional considerations. The situation can easily become more complex if an organization operates in multiple national contexts, since definitions in different national legal texts might differ from one country to another. Furthermore, an organization might be subject to multiple frameworks of rules if its collaborations with partners span national borders. For example, in one program that D-tree supports which is also classified as a research project, we must comply with:
-
two sets of data protection rules from separate institutional review boards,
-
the European Union’s General Data Protection Regulation (GDPR), and
-
organizational data protection guidelines from various partners.
Frameworks like the GDPR, as well as HIPAA in the United States, have been created in order to address the ambiguity surrounding national and international guidelines and laws; however, these frameworks are complex and have been written for stakeholders who have had considerable exposure to technology and relatively mature digitalized processes.
(v) Low digital literacy stands as a substantial barrier between policies and users.
In our programs, we often work with Community Health Workers (CHWs) and other frontline health workers who have low or no digital literacy—for some, it is the first time they have ever used a digital device. To make matters even more complex, these same users are then tasked with explaining critical digital concepts to the people they serve, who often have no exposure at all to intricate digital technology.
This poses a significant challenge in maintaining responsible and ethical data practices. Privacy, for example, becomes very abstract and difficult to understand in a digital setting; not just because of technological barriers, but also because some non-digital cultures might hold notions of privacy that are quite different from those held by system designers and other digitally-proficient stakeholders. A CHW would then need to get free, prior and informed consent from a client to enroll him into a digital health program in which personal data is collected—a difficult feat if the CHW doesn’t have a good grasp on the guidelines in the first place.
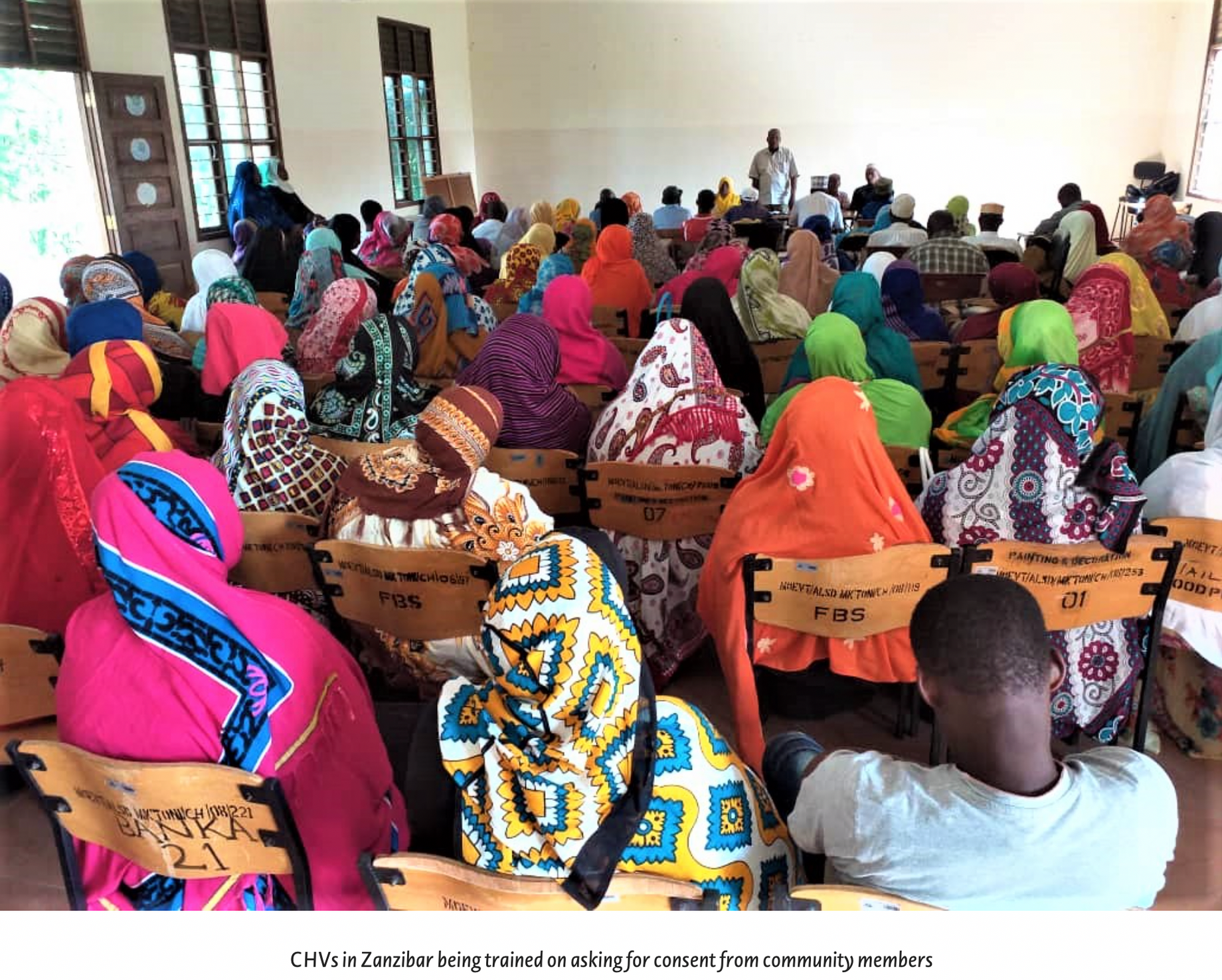
Data protection and privacy in practice
Because of the considerable complexities surrounding DPP as outlined above, D-tree strives to address DPP considerations throughout all phases of program or systems design, rather than considering DPP only after the digital system has been built. One of the ways we do this is by employing a human-centered design process that actively involves a variety of stakeholder representatives, including, but not limited to, the users themselves.
For example, when we started designing the health services to be offered as part of the Jamii ni Afya program in Zanzibar, one of the foundational principles we relied on was data minimization: a process of limiting data collection to what is essentially required to deliver services. Data minimization is not only beneficial to privacy, but also generally reduces system complexity.
Limiting data collection might sound counter-intuitive, as people sometimes have the reflex to gather as much information as possible because “you never know whether you might need it,” and “we could use it for additional analyses later on.” However, gathering data without a clear objective or previously-defined method for data analysis seldom yields quality data that can be used for meaningful analysis.
In the context of the Jamii ni Afya program in Zanzibar, this meant that a CHW’s visit would not include cumbersome collection of large amounts of data—data that that might have been interesting for certain purposes, but which would not necessarily have helped to increase the health of Zanzibaris.
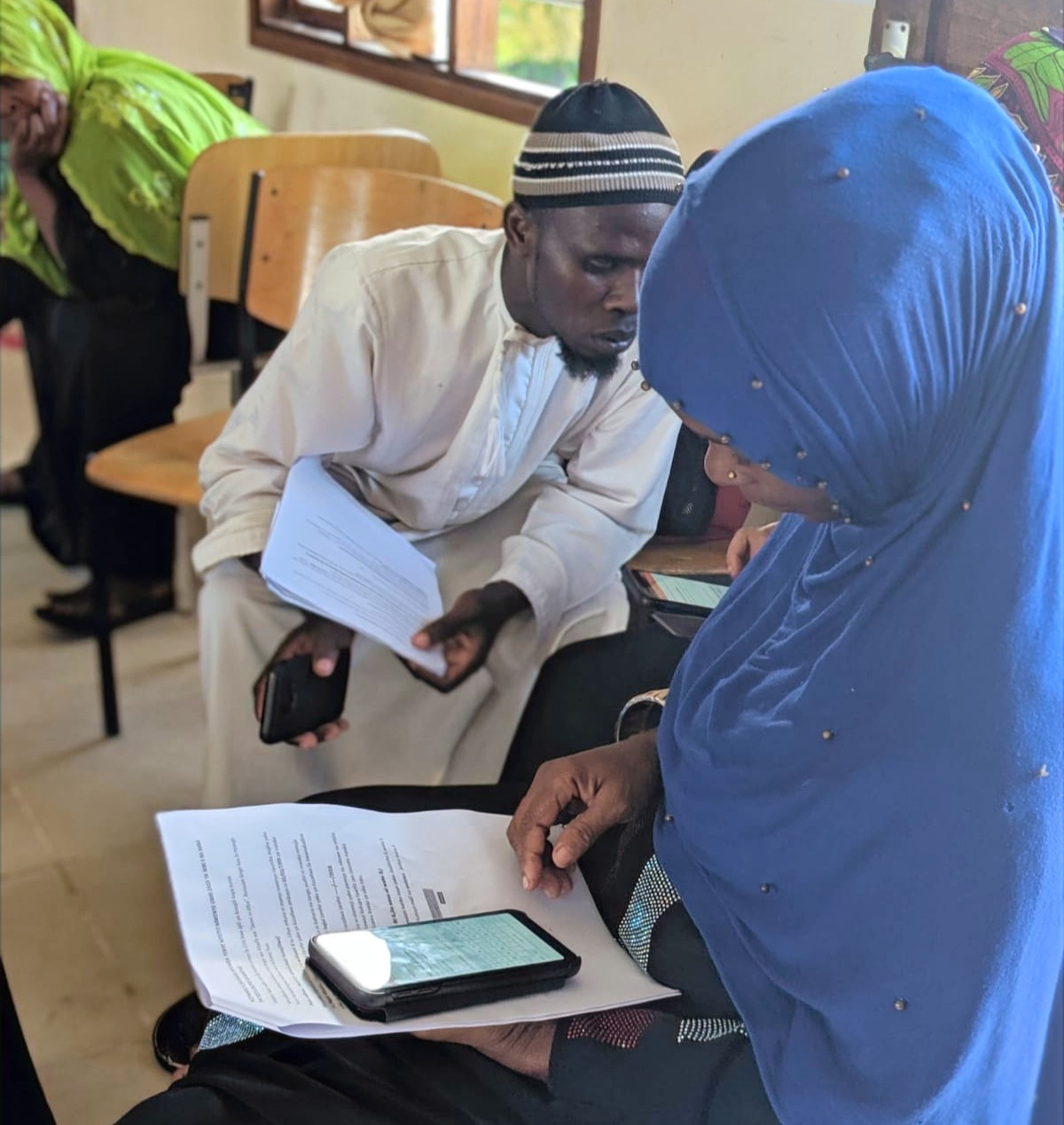
Data minimization case study: The rotavirus vaccine
To understand the principle of data minimization, it can be useful to look at a single data point collected in a system. Take, for example, our approach to rotavirus vaccinations in the Jamii ni Afya program. If we focus only on the date that the first rotavirus vaccine was administered to a child, we would first ask ourselves why this data point is important to collect. Is it required:
-
to enable a CHW to conduct his work?
-
to help a district health manager assess the immunization status in her district?
-
to facilitate central, nationwide immunization reporting?
-
to satisfy donor requirements?
If the only reason to collect a data point is to satisfy donor requirements, this could be a sign of poor program design. This would be especially true in a program like Jamii ni Afya, which aims to strengthen the health system in Zanzibar.
We avoid this by including relevant local stakeholders into discussions even before submitting any donor or funding agency. If a government’s objective is to increase rotavirus immunization coverage, a data point such as, “Received first rotavirus dose? [Yes/No]” might be sufficient. On the other hand, as is the case in Zanzibar with relatively good vaccine coverage, the Ministry of Health might be interested in evaluating and increasing the timeliness of vaccination. This is a case in which more detailed information is appropriate; therefore, in the Jamii ni Afya system, we capture the exact vaccination date instead of just asking whether or not the vaccine was received.
Both the questions we ask, and the solutions we deploy, depend on the on-the-ground realities and the local context.
Thus, for the same data point, we might get different answers in different contexts. In a different program with a different set of stakeholders, we might even get different questions altogether.
The example of data minimization around the single data point of a rotavirus vaccination exemplifies the complexity surrounding—and the critical importance of—both the DPP thought process and the related considerations that go into responsibly developing a digital system. We started with the question of whether we really need to collect it and for what purpose, then touched on what exactly the data point should look like. In practice, we would also need to consider which users would have access to the data point. This example shows that both the questions we ask, and the solutions we deploy, depend on the on-the-ground realities and the local context of a given project; therefore, a participatory, human-centered design approach is required to responsibly incorporate DPP into the design of any system.
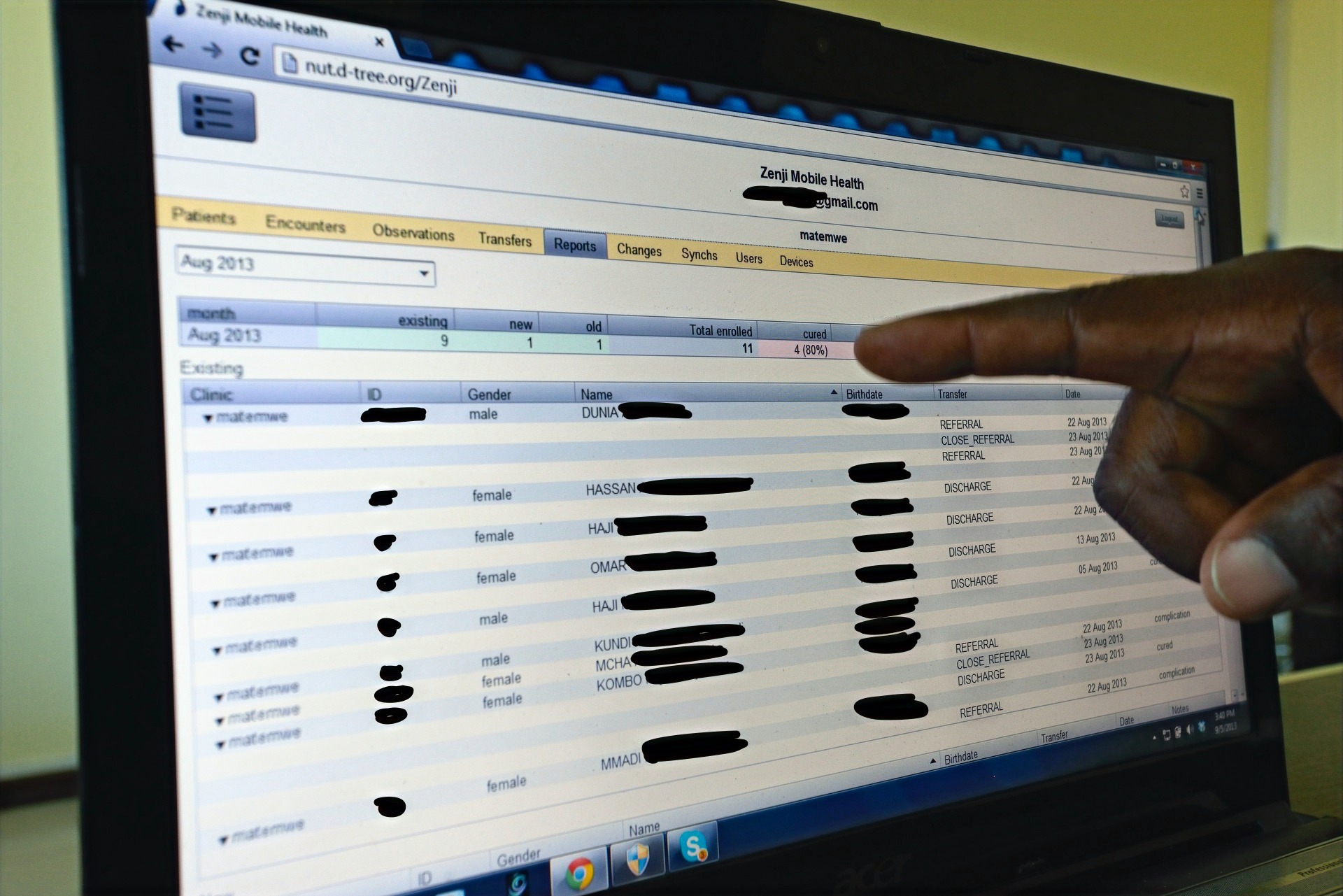
Beyond data minimization
While data minimization is certainly an important principle for DPP, it is by no means the only consideration. In designing the Jamii ni Afya program in Zanzibar around DPP, other elements included:
-
Data security and usability. Keeping data safe on the CHW devices and within the general system landscape required a balance between usability and security, as well as an acknowledgment that there is no perfect security. If the system enforced overly strict or complex CHW account passwords, for example, our CHWs might understandably record the password in a secondary location so as not to forget it—jeopardizing the account’s security. In this way, focusing too intently on security and neglecting usability runs the risk of users finding “workarounds” that actually compromise security.
-
Meaningful consent. This was an issue in part because notions of consent and privacy differ across cultures. For example, in a culture with a very strong community focus, we encountered a village leader who assured us that we “don’t need to worry about consent” because people would participate if he told them to do so. To us, this was not an acceptable form of consent, so we worked with that leader to understand the importance of individual informed consent and the right of any person to decline to participate.
-
Data de-identification. In order to share our findings with external research partners, we first had to ensure that our participants’ personal identities could not be revealed from the data—a process called “data de-identification.” The technical aspects of this process are only part of the story; even with clear data governance and strong de-identification protocols in place, it is also crucial to discuss which data points, if any, are culturally or politically acceptable to share.
Each of these topics serves to illustrate the complexities of collecting individual-level data. Nonetheless, individual datasets have enormous potential for public health and for medicine, as they significantly improve patient care and enable powerful analysis techniques that would not be possible with aggregate data.
Although DPP has been on the agendas of the digital health and digital development fields for quite some time, new technologies, as well as programs like Jamii ni Afya that generate new insights, keep challenging our thinking around DPP and push us to find appropriate solutions.
Throughout all of our programs, despite the sheer volume and complexity of information surrounding DPP, our most important priorities are:
-
Protecting the privacy and rights of the people in the communities we serve.
-
Complying with policies and regulations of international oversight bodies, of the ministries of health in the countries where we work, and of our development and implementation partners.
Within these boundaries, there is great potential to utilize de-identified data responsibly to benefit our programs, the health systems we work in, and global learning. We acknowledge that we, together with the broader field, need to try to tap into that potential. At D-tree, we aim to do this in an inclusive way—by leveraging our strong expertise in privacy and design, by engaging stakeholders in all phases, and by exchanging knowledge and best practices with partner organizations to benefit from and contribute to the field of global digital health.